Currently, the whole world is affected by COVID-19 pandemic. Wearing a face mask will help prevent the spread of infection and prevent the individual from contracting any airborne infectious germs.
When someone coughs, talks sneezes they could release germs into the air that may infect others nearby. Face masks are part of an infection control strategy to eliminate cross-contamination.
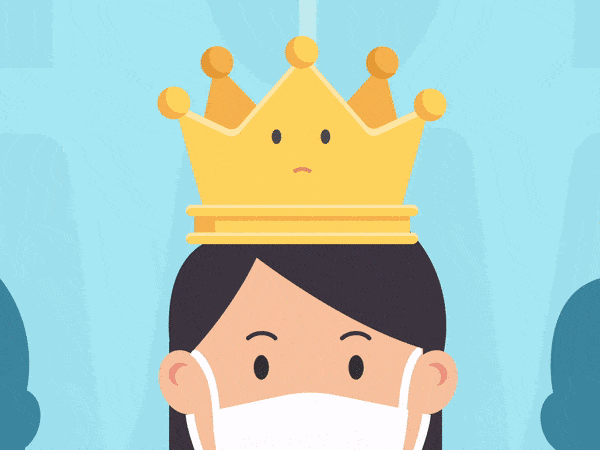
This Face Mask Detection system built with OpenCV, and TensorFlow using Deep Learning and Computer Vision detects face masks in real-time video streams.
Two-phase COVID-19 face mask detector
In order to train a custom face mask detector, the project is divided into two distinct phases, each with its own respective sub-steps :
- Training: Here we’ll load our face mask detection dataset from disk, train a model (using TensorFlow) on this dataset, and then serializing the face mask detector to disk
- Deployment: Once the face mask detector is trained, we can then load the mask detector, performing face detection, and then classifying each face as with_mask or without_mask.
Dataset
Dataset Used for this project is Face Mask Detection Data from Kaggle. It is a data of 3833 images belonging to two classes:
- with_mask: 1915 images
without_mask
: 1918 images
Implementing COVID-19 face mask detector training script
To train a classifier to automatically detect whether a person is wearing a mask or not, we’ll be fine-tuning the MobileNet V2 architecture, a highly efficient architecture that can be applied to embedded devices with limited computational capacity (ex., Raspberry Pi, Google Coral, NVIDIA Jetson Nano, etc.).
In order to proceed we will :
1. Import all the dependencies and required libraries.
2. Load and label the images in the Dataset.
3. Prepare the inputs for the Model
4. Construct and compile the Model
5. Train the Model and make predictions on the testing set
6. Plot the training loss and accuracy
Implementing our COVID-19 face mask detector in real-time video streams with OpenCV
1. Define face detection/mask prediction function
2. Load the Face Detector Model and Facemask Detector Model
3. Initialize the webcam video stream and detect the mask!
Cainvas Notebook: Face Mask Detector
YouTube demo : COVID-19: Face Mask Detector
Thank you.
Written by: Ritik Bompilwar
Also Read: Covid-19 Detection